What is TinyML?
Imagine having to manage heavy equipment or machinery that operates in a remote mountain area. Internet coverage is spotty at best and having an on-site technician to constantly monitor the equipment is out of the question. TinyML solves these issues as it enables real-time, remote monitoring of assets. Processing takes place closer to the equipment and reduces reliance on a steady cloud connection.
What distinguishes TinyML from ML?
Machine Learning has been around for a long time, but it is less often associated with hardware. ML and hardware are often connected with the cloud. TinyML is a novel approach to edge computing where machine learning models can be deployed and trained on edge devices.
TinyML is a type of machine learning where Artificial Intelligence is introduced into tiny hardware components. AI and intelligent gadgets are combined in these projects.
With tinyML, advanced techniques are used to train networks on devices and minimize their size. A big advantage is that there is no need to upload data to the cloud, which eliminates the additional delay in analyzing it.
What Exactly Is Edge Analysis?
Edge analysis is performed at non-central components of the system. Instead of relying on a central location miles away, insights are gathered closer to the device where: the site of data collection is. This includes sensors, switches, and numerous connected devices. Analysis literally takes place at the edge.
As IoT technologies permeate industry, the volume of data collected for analysis increases exponentially. This then needs to be transferred from the edge to the central processing point. Transferring insights between the two points takes valuable time. Edge analytics delivers decentralized insights and shortens this delay.
The current approach to edge analytics involves ML models trained in the cloud. In this method, latency is inevitable, and the system is prone to encountering privacy issues.
What are the Advantages of TinyML?
-
- Fast inference and low latency: On- device analysis means that data does not need to be sent to a server. The data is processed on the device, eliminating system latency.
- No internet connection required: Since the data is not constantly transmitted to the server, the smart edge devices are able to make inferences in the absence of an internet connection.
- Microcontrollers are energy efficient: They can run on batteries for an extended period. This saves energy and has the added benefit of being cost effective.
- Secure Data and Privacy: Data is kept at the edge and is not shared with other users or websites. This means that the risk of sensitive data being compromised is greatly reduced.
Apart from bringing AI to millions of everyday devices such as ATM’s, cell phones, calculators, and printers, tinyML offers important advantages. The graph below shows the growing interest in tinyML as more and more industries continue to recognize the value it offers.
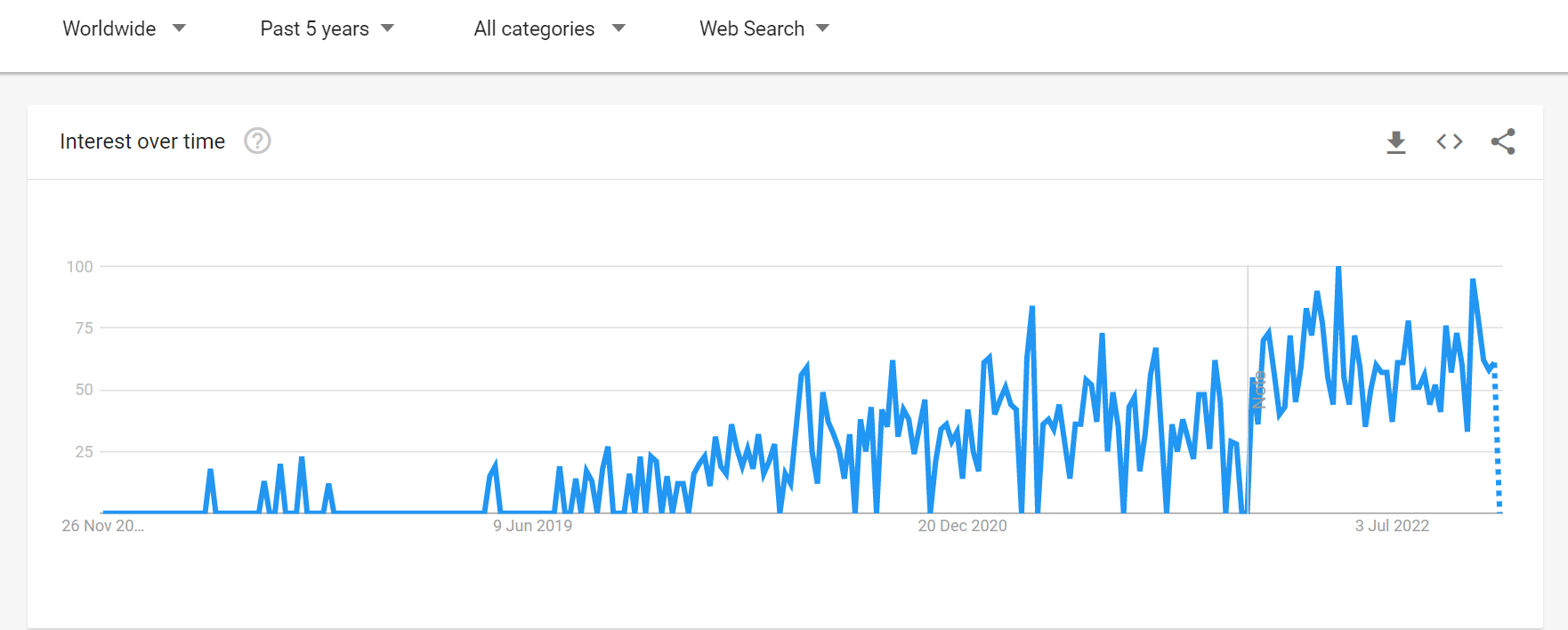
Source: Google Trends
Applications and Industries
TinyML has the ability to revolutionize situations where IoT data is used with reduced latency and improved data protection. Industries that can benefit from TinyML include:
- Agriculture: TinyML devices gather real-time data by monitoring crops or livestock. This allows farmers to intervene in a timely manner or adjust variables to prevent waste.
- Civil Engineering: With TinyML and edge AI, insights are gathered at the site where vibrations are created. This makes anomaly detection more efficient and allows for real-time alerts.
- Equipment: TinyML-powered predictive maintenance can reduce the downtime and costs associated with equipment failure.
- Healthcare: TinyML devices enable real-time health monitoring, which help to provide better and more personalized patient care
- Retail: TinyML is a practical way to monitor inventories and send alerts and helps to prevent out-of-stock situations.
- Personal Protective Equipment: Connected safety gear for emergency teams is a necessity. TinyML comes into play as embedded algorithms help detect environmental dangers such as contaminants or man-down situations.
The Future of TinyML
According to ABI Research, TinyML integration is estimated to hit 2.5 billion by 2030. This guarantees a boost to the economy, providing cost-effective, intelligent products. TinyML promises to revolutionize IoT devices and their applications by offering a sustainable, secure alternative.